I remember attending the Society of Judgment and Decision Making conference in Chicago in 2009. This society brings together psychologists who, following the footsteps of Kahneman and Tversky, have significantly influenced behavioural economics. The first talk I attended presented a cognitive “bias”; it was interesting. The second talk presented another cognitive bias, it was also interesting. The third and fourth talks followed suit. By that point, I started wondering how all these biases fit together. Were they even compatible?1
After about ten talks, I felt overwhelmed by the mountain of “effects” labelled as biases, with no overarching explanation in sight. It seemed to me that the field had turned into a kind of gold rush for cognitive flaws. For doctoral students starting their careers, discovering a new bias appeared almost essential to producing a successful thesis. For later career researchers, quirky and unexpected findings about cognition were a pathway to tenure at prestigious universities, lucrative book deals, and public recognition.
More than a decade later, the replication crisis and high-profile cases of fraud in the behavioural sciences have exposed some of the negative consequences of these incentives.2
The swing back of the pendulum
The focus on biases was, in some ways, understandable. In the mid-20th century, economists developed formal theories of rational behaviour that depicted humans as homo economicus: Spock-like figures—exceptionally intelligent and emotionally detached.
In this context, behavioural economics largely emerged as an “undoing project,” systematically bringing economists back down to earth (Lewis, 2016).3 This research program was highly successful because the homo economicus model was demonstrably unrealistic. However, in the pursuit of biases and psychological limitations, the pendulum seems to have swung too far. The psychologist Gerd Gigerenzer (1991) famously accused the field of assuming that people are dumb. In response, Gilovitch and Griffin admitted that even though it was not a foundational assumption in heuristics-and-biases research, it was effectively how the field operated in practice.
There is, however, one version of this critique to which researchers in the heuristics and biases tradition must plead ‘no contest’ or even ‘guilty’. This is the criticism that studies in this tradition have paid scant attention to assessing the overall ecological validity of heuristic processes. - Gilovich and Griffin (2002)
The seemingly endless list of newly discovered biases and cognitive flaws eventually pointed to a deeper issue: surely, we cannot be that bad at making decisions!
After decades of focusing on individuals’ cognitive limitations, there is now a renewed interest in explanations that highlight the efficient aspects of our cognition rather than its flaws.
Over the last decade or so, the pendulum has started to swing again toward some form of adaptiveness, or at least implicit purposefulness, in human cognition. - Benabou and Tirole (JEP, 2016)
Even Kahneman himself observed this shift. In an interview given shortly before his passing, he reflected:
I expect everything that I have done to be overwritten. It's nothing of eternal value. I am surprised that the work that Amos and I did has survived for half a century. That's remarkable. [...] I am seeing that happen. I am old enough and seeing the beginning of the overwriting. The future is going to be different. There is going to be, it seems in psychology, a return to rationality. - Kahneman (2024)
It is from that perspective that I wrote the book Optimally Irrational as a counterpoint to the prevailing emphasis on human limitations.
Reverse engineering
Seeking good reasons behind our behaviours makes sense because we are not here by chance alone. Every living individual today is the culmination of an extremely long lineage of ancestors who succeeded in surviving and reproducing. From cell biology to neuroscience, evolution has produced incredibly complex and efficient designs for survival and reproduction. This naturally has implications for our understanding of psychology:
Evolution pushes all animals towards the maximization of [fitness], and the evidence we have is that it pushes hard … nervous systems are pushed to effectively maximize fitness with efficient neural circuitry. - Glimcher (2010)
Although evolution is an impersonal process, it leads to organisms that appear as if they were purposefully designed. In The Blind Watchmaker, Dawkins highlights this point:
Biology is the study of complicated things that give the appearance of having been designed for a purpose. - Dawkins (1986)
Studying human psychology is, therefore, an exercise in reverse engineering.4 When encountering an unexplained behaviour, one should aim to uncover the potential rationale behind it.
The idea that an impersonal process like evolution can produce efficient “designs” may feel counterintuitive. As Dawkins noted, this is because our intuitions are not designed to grasp processes occurring on vastly different timescales from our own:
Another way in which we seem predisposed to disbelieve Darwinism is that our brains are built to deal with events on radically different timescales from those that characterize evolutionary change. We are equipped to appreciate processes that take seconds, minutes, years or, at most, decades to complete. Darwinism is a theory of cumulative processes so slow that they take between thousands and millions of decades to complete. All our intuitive judgements of what is probable turn out to be wrong by many orders of magnitude. Our well-tuned apparatus of scepticism and subjective probability-theory misfires by huge margins, because it is tuned—ironically, by evolution itself— to work within a lifetime of a few decades. - Dawkins (1986)5
Answering criticisms
No predictions
A common criticism of evolutionary theory is that it does not make predictions and only offers ex-post explanations. This criticism is misguided.
Evolutionary explanations rest on the principle that the features of life are shaped by fitness maximisation. By applying this principle to as-yet-unobserved aspects of life, one can test its predictive power. In the behavioural sciences, evolutionary psychologists frequently use this approach to predict behaviours. One example is the testing of Hamilton’s rule, which posits that people are more inclined to help kin in proportion to their degree of relatedness. Empirical studies have supported these predictions (Hames, 1987; Jones, 2000).
Moreover, evolutionary explanations about why certain traits exist impose constraints on how they should function. Regarding human cognition, Laith Al-Shawaf (2024) observed:
A hypothesis about the evolved function of an information-processing system leads to predictions about how the system must be structured to achieve that function.6
Thus, evolutionary explanations generate empirically testable predictions about psychological features. In fact, much of evolutionary behavioural science is built on testing hypotheses about behaviour using empirical data.
Just so stories
Another popular criticism of evolutionary explanations is that they are “just so” stories. The expression comes from the biologist Stephen Jay Gould who accused his colleagues of proposing explanations that look like Rudyard Kipling’s tale of the leopard who got painted spots on his fur “just so” he could be better camouflaged.
This critique has two aspects. The first concerns the explanation of features of organisms purely by their function, a hallmark of the reverse-engineering approach. Gould and Lewontin (1979) elaborated on this critique in a seminal paper, where they criticised the “adaptationist” programme. They argued that this approach often overlooks the broader constraints affecting an organism as a whole. Key among their points was that “architectural constraints” can significantly shape evolution, preventing organisms from being ideally adapted to their environments.7 They also noted that some traits may simply be by-products of evolutionary pressures acting on other parts of the organism.
Modern evolutionary science has taken these critiques into account and is more cautious than in the past. For example, Jeff Ely (2011) modelled how architectural constraints due to path dependency can lead to “kludged” designs—imperfect solutions shaped by evolutionary history.
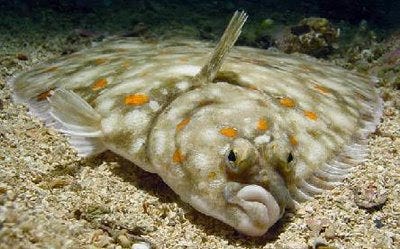
The second aspect of the criticism concerns the plausibility of evolutionary “stories.” Some explanations in the past may have been accepted too readily based on their surface plausibility. However, this does not reflect how evolutionary science is conducted today. Modern evolutionary theories rely on a few core principles that yield a wide range of predictions. These principles, being broadly accepted, constrain researchers’ ability to concoct flexible, ad hoc explanations.8
Evolution cannot product perfect designs, only “good enough” ones
Another critique suggests that evolution does not optimise but merely “satisfices,” a term introduced by Herbert Simon (1955). According to this view, evolutionary designs are simply “good enough” for survival and reproduction. However, this idea misrepresents the competitive nature of evolution. As Dawkins argued in The Extended Phenotype:
The trouble with satisficing as a concept is that it completely leaves out the competitive element which is fundamental to all life. In Gore Vidal’s words: ‘It is not enough to succeed. Others must fail.’ - Dawkins (1982)
Natural selection is a competitive process and hence there is a pressure not just for good enough designs but for the best designs. Best here does not mean “perfect”. Evolution faces trade-offs and perfection is often too costly to achieve to be worth it. Instead, “best” means optimal under the biological constraints faced by the evolutionary process.9 Part of the reverse-engineering approach is, therefore, to recognise which constraints and trade-offs our current features may be good answers to.
Why is it needed?
Some behavioural scientists argue that evolutionary explanations are unnecessary for understanding human behaviour. They say one just has to study behaviour that we can observe in the present. This claim is puzzling—it is akin to saying we do not need physicists to study the Big Bang because we can simply observe the universe as it exists now.
An evolutionary framework offers practical benefits, including serving as an epistemic prophylaxis: a safeguard against flawed explanations. For example, psychologists often attempt to explain behaviour by the mental states they generate. Consider overconfidence: it has been explained as stemming from the fact that people enjoy thinking they are better than they are. However, overconfidence comes with costs like paying the price for taking too much risk. If overconfidence provides benefits only as “good feelings” and imposes real-world costs, how could these “feelings” have been selected for in the first place?10
The evolutionary biogist Robert Trivers resolved this contradiction with an alternative explanation: overconfidence may have evolved because it provides social advantages, such as impressing others with perceived competence (Trivers, 1976; von Hippel & Trivers, 2011). Recent experimental studies support this view (Schwardmann et al., 2019; Soldà et al., 2020).
An evolutionary perspective is useful for distinguishing between viable and non-viable explanations of behaviour.
Way forward
Renewed interest in foundational and adaptive explanations
Reflecting the pendulum swinging back, behavioural scientists are increasingly seeking foundational explanations that provide a unified understanding of and sound reasons for observed behaviours.
In economics, several strands of research illustrate this shift. The theory of rational inattention posits that the costs of acquiring information explain why individuals gather limited information, making some degree of error essentially optimal (Maćkowiak et al., 2023). Research on cognitive uncertainty suggests that many mistakes arise because people are unsure about what to believe, and belief updating is challenging when information is complex (Enke, 2024). Studies on the evolutionary foundations of preferences explore how cognitive limitations may reflect effective solutions for managing decision-making, given biological constraints (Robson and Samuelson, 2011).11
In psychology, the framework of resource rationality similarly seeks to understand cognitive limitations as optimal adaptations to the resource constraints faced by the brain (Lieder and Griffiths, 2020). And in neuroscience, the research following the Bayesian brain hypothesis assumes that our brain is quite a remarkable computing machine solving challenging information processing problems (Knill and Pouget, 2004; Yon and Frith, 2021).
Renewed justification for the use of optimisation tools
Behavioural economics was founded on a critique of the homo economicus model, which assumes individuals rationally solve complex problems in their heads. The pendulum is now swinging back towards the view that people may, in fact, solve complex mathematical problems when making decisions. While individuals might struggle with novel problems, evolution could have selected cognitive programs capable of addressing problems frequently encountered by our ancestors:
An animal may be pre-programmed in such a way that it behaves as if it had made a complicated calculation. - Dawkins (1976)
Moreover, evolution has equipped us with the ability to learn when faced with novel challenges. This capacity allows us to approximate optimal solutions through trial and error, and mental simulations.12
As a consequence of both these innate and learned abilities, I argued in Optimally Irrational (2023) that the mathematical tools of optimisation and game theory can be used to make sense of observed behaviour. But the use of these tools should differ somewhat from how they were used in the era of the homo-economicus. While economists assumed individuals to face no cognitive constraint, an adaptive approach must account for the constraints—such as the biological limitations of the nervous system—that influence decision-making.
Systemic flaws must have an explanation
In the literature on cognitive biases, deviations from rational behaviour have often been labelled as “effects,” providing a superficial sense of explanation without addressing the underlying causes. An adaptive perspective challenges this approach by demanding deeper inquiry into the origins of behavioural flaws.
From this perspective, behavioural scientists should aim to uncover the sources of these apparent flaws. In some cases, flaws may result from architectural constraints inherent to cognitive or biological systems. In other instances, they could stem from evolutionary mismatches—behaviours that were advantageous in ancestral environments but are less suited to modern conditions. Often, however, what appears to be behavioural flaws may actually be optimal solutions given the constraints faced by evolution.
Humans are the product of a long process of evolution. Our ancestors have been selected over eons for the quality of their everyday decisions, big and small. For that reason, it makes sense to start the study of behaviour by asking, “What could be the good reasons behind this behaviour?” In many cases, what initially seems like a bias or a flaw is revealed as a good solution when the complexity of the decisions we have to make and the cost of solving are taken into account.
This argument does not imply that all behaviour has an adaptive explanation or that cognitive flaws do not exist. Instead, it suggests a methodological approach: when encountering unexpected or puzzling behaviours, researchers should first consider the possibility that they may not fully understand the underlying reasons. They should investigate potential explanations rather than prematurely labelling the behaviour as a mistake that could be easily avoided.
This is the first of a series of three posts on the value of taking an evolutionary perspective when trying to understand human behaviour.
References
Al-Shawaf, L., 2024. Levels of Analysis and Explanatory Progress in Psychology: Integrating Frameworks from Biology and Cognitive Science for a More Comprehensive Science of the Mind. Psychological Review.
Bénabou, R. and Tirole, J., 2016. Mindful economics: The production, consumption, and value of beliefs. Journal of Economic Perspectives, 30(3), pp.141-164.
Dawkins, R., 1976. The Selfish Gene. Oxford: Oxford University Press.
Dawkins, R., 1982. The Extended Phenotype: The Long Reach of the Gene. Oxford University Press.
Dawkins, R., 1986. The Blind Watchmaker: Why the Evidence of Evolution Reveals a Universe Without Design. WW Norton & Company.
Ely, J.C., 2011. Kludged. American Economic Journal: Microeconomics, 3(3), pp.210-231.
Enke, B., 2024. The Cognitive Turn in Behavioral Economics. Mimeo Harvard.
Gilovich, T. and Griffin, D., 2002. Introduction: Heuristics and Biases: Then and Now. In: T. Gilovich, D. Griffin, and D. Kahneman, eds. Heuristics and Biases: The Psychology of Intuitive Judgment. Cambridge: Cambridge University Press, pp.1-18.
Glimcher, P.W., 2010. Foundations of Neuroeconomic Analysis. Oxford University Press.
Gould, S.J., 1978. Sociobiology: The Art of Storytelling. New Scientist, 80(1129), pp.530-533.
Gould, S.J. and Lewontin, R.C., 1979. The Spandrels of San Marco and the Panglossian Paradigm: A Critique of the Adaptationist Programme. Proceedings of the Royal Society of London. Series B. Biological Sciences, 205(1161), pp.581–598.
Hames, R., 1987. Relatedness and Garden Labor Exchange Among the Ye’kwana. Ethology and Sociobiology, 8(4), pp.354–392.
Jones, J.H., 2000. Kin Selection and the Evolution of Human Altruism. In: L. Cronk, N. Chagnon, and W. Irons, eds. Adaptation and Human Behavior: An Anthropological Perspective. New York: Aldine de Gruyter, pp.261–282.
Kipling, R., 1902. Just So Stories for Little Children. London: Macmillan and Co.
Knill, D.C. and Pouget, A., 2004. The Bayesian brain: the role of uncertainty in neural coding and computation. TRENDS in Neurosciences, 27(12), pp.712-719.
Kuhn, T.S., 1962. The Structure of Scientific Revolutions. Chicago: University of Chicago Press.
Lewis, M., 2016. The Undoing Project: A Friendship That Changed the World. Penguin UK.
Lieder, F. and Griffiths, T.L., 2020. Resource-Rational Analysis: Understanding Human Cognition as the Optimal Use of Limited Computational Resources. Behavioral and Brain Sciences, 43, p.e1.
Marblestone, A.H., Wayne, G. and Kording, K.P., 2016. Toward an Integration of Deep Learning and Neuroscience. Frontiers in Computational Neuroscience, 10, p.215943.
Maćkowiak, B., Matějka, F. and Wiederholt, M., 2023. Rational Inattention: A Review. Journal of Economic Literature, 61(1), pp.226-273.
Page, L., 2023. Optimally Irrational: The Good Reasons We Behave the Way We Do. Cambridge University Press.
Pigliucci, M., 2014. Between Holism and Reductionism: A Philosophical Primer on Emergence. Biological Journal of the Linnean Society, 112(2), pp.261-267.
Rieskamp, J., Busemeyer, J.R. and Mellers, B.A., 2006. Extending the Bounds of Rationality: Evidence and Theories of Preferential Choice. Journal of Economic Literature, 44(3), pp.631-661.
Robson, A.J. and Samuelson, L., 2011. The Evolutionary Foundations of Preferences. Handbook of Social Economics, 1, pp.221-310.
Simon, H.A., 1955. A Behavioral Model of Rational Choice. The Quarterly Journal of Economics, pp.99-118.
Soldà, A., Ke, C., Page, L. and Von Hippel, W., 2020. Strategically Delusional. Experimental Economics, 23, pp.604-631.
Thaler, R.H., 2015. Misbehaving: The Making of Behavioral Economics. WW Norton & Company.
Tooby, J. and Cosmides, L., 1992. The Psychological Foundations of Culture. The Adapted Mind: Evolutionary Psychology and the Generation of Culture, 19(1), pp.1-136.
Trivers, R.L., 1976. Preface. In: R. Dawkins, The Selfish Gene. Oxford: Oxford University Press.
Schwardmann, P. and Van der Weele, J., 2019. Deception and Self-Deception. Nature Human Behaviour, 3(10), pp.1055-1061.
Vandermassen, G., 2005. Who's Afraid of Charles Darwin?: Debating Feminism and Evolutionary Theory. Rowman & Littlefield Publishers.
Von Hippel, W. and Trivers, R., 2011. The Evolution and Psychology of Self-Deception. Behavioral and Brain Sciences, 34(1), pp.1-16.
Yon, D. and Frith, C.D., 2021. Precision and the Bayesian brain. Current Biology, 31(17), pp.R1026-R1032.
It is a fair question to ask when many separate models are presented to explain behaviour. Rieskamp et al. (2006) showed that two models proposed by Tversky to explain different biases were based on conflicting assumptions, meaning both could not be correct simultaneously.
The behavioural sciences faced a significant challenge during the replication crisis of the 2010s. Many high-profile “wow” findings that described strange and unexpected behavioural patterns failed to replicate under scrutiny. One well-known example is the “power posing” study by Amy Cuddy and her co-authors, which claimed that adopting a dominant pose—standing with hands on hips—boosted testosterone levels and confidence. Cuddy’s TED talk on the topic became one of the most viewed ever, amassing over 25 million views and offering what seemed like a simple life hack for success in interviews or dating. However, subsequent attempts to replicate the findings failed, and it emerged that questionable research practices, including p-hacking, had likely influenced the original results (see for instance this Data Colada post).
Even more troubling were cases of fraud by prominent researchers. Dan Ariely and Francesca Gino, both celebrated figures in behavioural science, faced allegations of fabricating data. Ironically, both had worked extensively on dishonesty, even co-authoring a paper arguing that dishonest individuals tend to be more creative. Evidence later suggested that they had independently manipulated data, even in a joint paper.
The stakes for producing surprising behavioural findings were high, with potential rewards including fame and financial gain. Dan Ariely became a household name with his popular book on behavioural science, in which he argued that humans are fundamentally irrational. By 2020, Francesca Gino was Harvard’s fifth-highest-paid employee, earning over $1 million annually, and commanded $50,000–$100,000 per speaking engagement.
Several behavioural economists have noted that these issues were primarily concentrated in psychology rather than behavioural economics, which typically resides within economics departments. Economics relies on a strong theoretical framework, making it less susceptible to accepting unexpected results without robust evidence. Nonetheless, behavioural economics has been influenced by the trend of focusing on cognitive biases. It has become significantly easier to publish papers proposing behavioural patterns as biases without further justification than it was two decades ago.
For the interested readers, I developed my views on these scandals and what they mean for behavioural science in this post.
Richard Thaler recalls how, early in his career, psychologists were incredulous at the standard economic view of homo economicus optimising consumption and savings over a lifetime. As he remarked, “Psychologists remained stunned in disbelief, wondering how their economics department colleagues could have such wacky views of human behavior” (Thaler, 2015).
At its core, the discovery of the design of human psychology and physiology is a problem in reverse engineering. - Tooby and Cosmides (1992)
As pointed by Laith Al-Shawaf, this approach aligns with classical questions in biology proposed by Tinbergen—looking for function—and by Marr—looking for the computational problems being solved.
Tinbergen’s function and Marr’s computational theory both center on what problem the system is “trying” to solve, what task the system is meant to handle, and why the system does what it does. - Al-Shawaf (2024)
A useful way to appreciate the timescale of evolution is to represent the timeline of life on Earth along a human arm. Hominids appeared around 6.5 million years ago. If 1,000 years is long, 6.5 million years is 6,500 times that duration! Still, that places early hominids only at the end of the fingertips on that picture.
Al-Shawaf gives the following example about the feeling of disgust:
The way one tests a hypothesis about the evolved function of disgust is via the many proximate predictions it yields about how disgust ought to work in the present. For example, the disease-avoidance hypothesis about the function of disgust predicts that disgust should be a universal emotion, that some stimuli should be universally disgusting, that these universally offensive substances should be pathogenic in nature, that we are more easily disgusted when we are sick or immunocompromised, that triggering disgust or pathogen salience will make people behave in ways that reduce the likelihood of infection, that disgust will be context-sensitive and downregulated toward one’s kin, that disgust will be characterized by a “better safe than sorry” error management bias, and that individuals who grow up in more pathogen-dense parts of the world will be more wary around other people. - Al-Shawaf (2024)
This aspect of their criticism belongs to the recurrent debate about reductionism (explaining complex things by their parts) versus holism (saying things must be explained as a whole) in science (Pigliucci, 2014).
Efforts to explain subjective satisfaction highlight the utility of minimal assumptions. Two constraints—subjective satisfaction as a bounded and noisy signal—suffice to generate the key features of Kahneman and Tversky’s Prospect Theory.
Any view of biological optimization that denies the existence of costs and trade-offs is doomed. - Richard Dawkins (1982)
One classic example is the speed-accuracy trade-off. A visual system must be precise enough to recognise objects but fast enough to react in time. Perfect accuracy could take too long—by the time you identify an animal nearby, it might already be out of reach (prey) or attacking you (predator). Optimal design balances speed and accuracy under such constraints
Evolutionary psychologist Griet Vandermassen gave other examples of flawed explanations: key ideas from psychoanalysis.
There is sometimes an incompatibility between our knowledge of how evolution works and the specific proximate causes proposed by social scientists. The Freudian Oedipus complex, for instance, could never have evolved as a fundamental part of human nature. Because of the reduced viability of offspring produced by incestuous mating, the trait would soon have died out. The Oedipus complex, one of the core presumptions of psychoanalytic theory, would never have been given any credence if anyone had considered the evolutionary fate of a trait that produced such incestuous desires in each and every child. The same goes for Freud’s proposition of the death instinct, and for many other psychoanalytic concepts as well. - Vandermassen (2005)
For the economist reader:
This approach differs from evolutionary game theory, which gained popularity in the 1990s and early 2000s. I recently asked Larry Samuelson—the current president of the Econometric Society and a leading figure in the research on the evolutionary foundations of economic preferences—why the interest in evolutionary game theory waned. He suggested it was—paradoxically— because the field had been largely successful. It provided clear answers to two key problems it was tasked with:
Is Nash equilibrium a good concept in the sense that an, given evolutionary dynamics would converge to an equilibrium? (Yes.)
Do evolutionary dynamics lead to specific Nash equilibria, refining the equilibrium concept? (No.)
Incidentally, these two answers mean than an adaptive approach is compatible with studying the Nash equilibria of social games without necessarily modelling their evolutionary dynamic.
I like how Dawkins puts it in the Selfish Gene:
One way for genes to solve the problem of making predictions in rather unpredictable environments is to build in a capacity for learning. - Dawkins (1976)
I agree with you. I had an email debate with Gary Klein and Daniel Ariely about this in 2009.
This is what I wrote then.
"I would like to use hyperbolic discounting is an instructive example. This is normally portrayed as irrational behaviour or a "bias" (i.e. a deviation from rationality). However in a highly unstable environment it is actually a rational response. For most of our history, human beings have lived in such unstable environments (and many still do). Within that context, it is rational. In a new context (say, the Western world between 1993 & 2007) it is "irrational". The problem is that the patterns of optimal behaviour that human beings develop collectively (what we might call "rationality") tend to lag changes in our environment. A "bias" is not fundamentally irrational, it is simply a piece of rational pattern recognition being deployed in the wrong context.
Classical economics reminds me of nothing so much as classical mechanics. They are approximations that work well in certain defined contexts (although I think that classical mechanics was more successful than classical economics). Classical mechanics might be useful but it is not helpful in all contexts - and attempts to save it required the deployment of "fudge factors" such as "aether". Classical mechanics has been superceded by quantum mechanics and relativity - which is not to say that it isn't still used when required. The use of "bias" strikes me as a very similar fudge factor to "aether".
I think that the empirical findings of Behavioural Economics will stand the test of time but I also believe that its theoretical underpinnings need to be rewritten in the light of Naturalistic Decision Making and other cognitive sciences. What I'd like is some "Grand Unified Theory" (maybe not so grand but certainly more unified) of human behaviour.
I'm kinda strayin' into evolutionary psychology* territory here. Is there a link between NDM, BE & evolutionary psychology? Should there be?"
How is saying that "Evolution cannot produce perfect designs, only 'good enough' ones" even a critique? To me, as someone familiar with genetic algorithms—models inspired by natural selection—it seems precisely how evolution operates. Evolution is an iterative, never-ending process that doesn't aim for perfection or an optimal endpoint. Every "design" is either destined for extinction (with overwhelming probability) or evolves into a new, more adaptive form. Critiquing evolution for not producing perfect designs feels like critiquing a river for not being straight—it's simply not how the system works.