Unpacking the modern science of happiness
How neuroscience and AI help us understand the elusiveness of happiness
In The Unbearable Lightness of Being, Milan Kundera offers these thought-provoking reflections on the human condition:
We can never know what to want, because, living only one life, we can neither compare it with our previous lives nor perfect it in our lives to come. […] There is no means of testing which decision is better, because there is no basis for comparison. We live everything as it comes, without warning, like an actor going on cold. - Kundera (1984)
There are two ways to interpret Kundera’s dilemma. One interpretation is that happiness is elusive: we don’t know what will make us happy (e.g., a high income or a meaningful job?).1 The other interpretation is that we know what would make us happy but are uncertain about how to achieve it (e.g., when choosing between job offers). In this post, I discuss how the modern science of happiness can help us answer these questions and address Kundera’s dilemma.
Pleasure and pains as optimally informative signals
Positive and negative hedonic feelings can be understood as signals meant to guide our decisions. From this perspective, our hedonic system—the cognitive processes that produce these signals—has been shaped by evolution for us to increase our fitness by following these cues. This idea is widely accepted in behavioural sciences. For example, Kahneman described the role of pleasure and pain as follows:
The adaptive functions of pleasure and pain can be deduced from the conditions under which these experiences are biologically programmed to occur. Pleasure is evidently a “go” signal, which guides the organism to continue important activities such as foreplay or consuming sweet, energy-rich food. Pain is a “stop” signal, which interrupts activities that are causing harm, such as placing weight on a wounded foot. - Kahneman, Wakker, Sarin (1997)
But what exactly are these hedonic feelings, and how are they determined? Over the past three decades, cognitive neuroscientists have expanded our understanding: hedonic feelings are not just useful but are optimally designed to be as useful as possible.
These feelings, whether positive (pleasure, satisfaction) or negative (pain, dissatisfaction), can be seen as subjective values. Evolutionary pressure has shaped these values to induce good decisions. As neuroscientist Read Montague pointed out:
In order to survive, mobile creatures must be able to value the resources available and the choices they can make to acquire those resources. […] Nature’s economic realities have selected for creatures that can value their intended actions quickly and accurately - Montague (2007)
Let’s consider what “optimal” hedonic feelings might look like. The challenge of assigning values to decisions to guide an agent toward optimal choices is similar to the problem computer scientists solve when designing artificial intelligence systems meant to operate on their own. One famous example is AlphaGo from Google DeepMind, which plays the game of Go and defeated world champion Lee Sedol in 2016.2
For complex tasks like Go, a program cannot be given a simple recipe with correct instructions to win. It must learn how to behave. The program achieves this by associating values with different actions based on the estimated rewards for each action. In Go, the reward is the likelihood of winning the game. Through extensive training, AlphaGo learned to associate values with various moves in different game positions, allowing it to choose the action with the highest value to maximise its chances of winning.3
Estimating the value of a move is not straightforward. After any move, numerous scenarios can unfold. Should we consider all possible outcomes or just a subset? The optimal solution is provided by the Bellman principle of optimality. When a program estimates the value of a move, it should only consider the scenario where it continues to make the best decisions at each future step. The picture below provides an intuition for this principle of optimality.
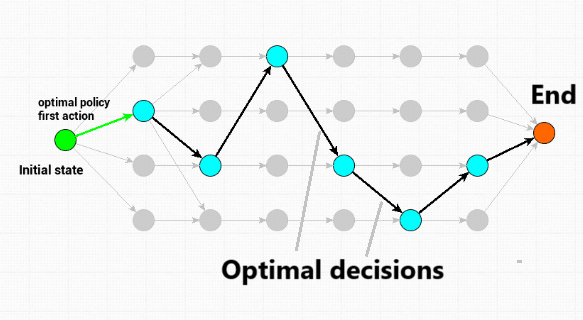
Computer scientists Sutton and Barto developed a key method for training AI systems to learn these values.4 The concept is simple: the program learns by observing deviations between its expectations of rewards and the actual rewards it receives. The difference between expected and actual rewards is used by the program to update its previous value estimate.
A major breakthrough in neuroscience occurred in the 1990s when researchers discovered that the brain appears to implement this same algorithm, which computer scientists use to train programs to make optimal decisions! This discovery emerged from empirical findings by neuroscientist Wolfram Schultz and the mathematical modelling of computational neuroscientists Read Montague, Peter Dayan, and Terry Sejnowski (1996).
Dopamine neurons, which are responsible for encoding “rewards,” do not simply respond to rewards; they encode the prediction errors between what the brain expects and what it experiences. In a classic experiment, Schultz observed that a monkey's dopamine neurons fired when it received orange juice. However, if a light signalled the arrival of the juice, the neurons fired in response to the light (the surprise) and not the juice. If the juice failed to appear after the light, the neurons' firing decreased, indicating frustration from an unmet expectation.
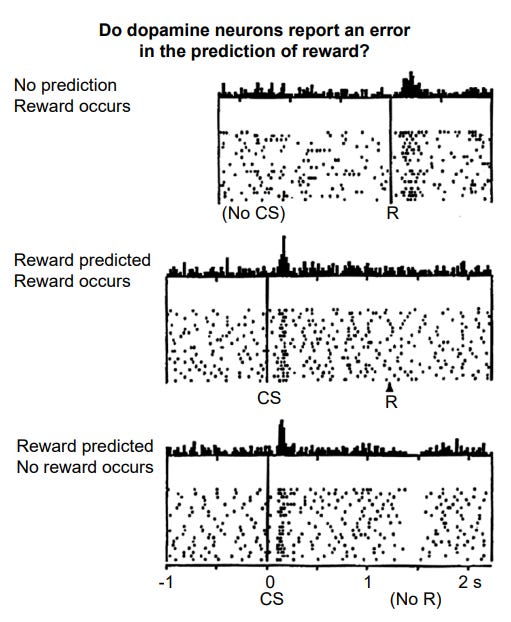
From that perspective, while our brain encodes the values of each choice we may face in expectation, the hedonic feelings we experience are only prediction errors—positive and negative surprises relative to these expectations.5
This insight provides the first answer to Kundera’s dilemma. It is no wonder we struggle to know how to be happy. Happy feelings arise from positive surprises relative to our expectations. Surprises are not, by definition, something we can achieve in a stable and predictable way. If we consistently experience positive outcomes, we begin to expect them, and the associated surprise—and thus happiness—diminishes.
The different types of rewards guiding our decisions
This also explains how we make decisions. Through repeated experiences, our hedonic system learns the expected value of different choices. In novel situations, our brains can use mental models of the world to estimate the likely value of various options. For example, if you’re considering jumping across a stream, you can simulate the action in your head to estimate whether it’s a good idea.
Primary rewards
In this decision-making process, the brain aims to maximise rewards. But what are these rewards? Some are linked to fundamental states that are important for survival and reproduction. We need energy (food), water and safety. Signals associated with these essential needs are known as primary rewards. In a social species like ours, having social support (family, friends) is also fundamental. Therefore, we experience social connections as primary rewards too. Social status, our standing in a group, also shapes our chances of success. As a result, we can expect it to act as a primary reward.
Status, like food and water, has value and is therefore rewarding to humans. -Montague (2007)
Secondary rewards
While these primary rewards are useful, we face contexts too specific for evolution to have generated perfectly calibrated rewards for every possible decision in every possible context (e.g. for the decisions of an Inuit in the Artic circle and the decisions of a Yanomani in the Amazon jungle). A solution is for evolution to have given us the ability to learn what actions are likely to generate the primary rewards associated with fundamental needs. As Richard Dawkins stated in The Selfish Gene.
One way for genes to solve the problem of making predictions in rather unpredictable environments is to build in a capacity for learning. Here the program may take the form of the following instructions to the survival machine: ‘Here is a list of things defined as rewarding: sweet taste in the mouth, orgasm, mild temperature, smiling child. And here is a list of nasty things: various sorts of pain, nausea, empty stomach, screaming child. If you should happen to do something that is followed by one of the nasty things, don't do it again, but on the other hand repeat anything that is followed by one of the nice things.’ - Dawkins (1976)
Through this learning process, we develop preferences for things that didn’t exist in the past, such as money, sports, or social media “likes”. These secondary rewards are learned associations with primary rewards like food (money), health (sports), and status (money and likes). The convergence between neuroscience and artificial intelligence I described above suggests that these secondary rewards are learned to approximate the optimal way to accumulate primary rewards.6
Primary rewards: circling back
Returning to primary rewards, the literature in cognitive neuroscience assumes that these values evolved to guide us in the right direction. But we can go further. Evolution itself functions as a learning process, selecting values that best guide decision-making.7 Our primary rewards, therefore, should respect the Bellman principle of optimality, reflecting the best-estimated values for a given state, considering all the actions we may take in the future. The pleasures and pains we experience from eating, drinking and other actions associated with primary rewards should be optimally designed to guide us towards survival and reproduction.8
There is only one twist. These values have been calibrated in an ancestral environment that is very different from our modern one. This can lead to predictable mismatches. For instance, we like energy-rich food more than necessary, given that it is now plentiful.
So how does happiness work? Our primary rewards are hardcoded values designed to optimally guide us towards outcomes beneficial for our fitness. Secondary rewards are values that we learn during our lives to optimally guide us towards acquiring these primary rewards in the specific contexts we face. In both cases, our hedonic system only generates hedonic feelings when our experiences differ from expectations, making the pursuit of lasting happiness elusive.
With this understanding of happiness, we can fully address Kundera’s dilemma. Kundera is correct that we may have imperfect information about what we should do for big and rare decisions. But, for most decisions in our life, our hedonic system helps us make good choices. We inherit through this system the insights from evolution embedded in the primary rewards that guide our decisions every day (directly and indirectly through secondary rewards). Even though we live only one life, our decisions are shaped by the experience of the countless lives of our ancestors.
This post is part of a series on happiness. I am going to end this series with a few posts on big questions about happiness. In my next post, I’ll discuss the different types of “Good”.
References
Berridge, K.C. and Kringelbach, M.L., 2015. Pleasure systems in the brain. Neuron, 86(3), pp.646-664.
Dawkins, R., 1976. The Selfish Gene. Oxford University Press.
Dayan, P., 2022. “Liking” as an early and editable draft of long-run affective value. PLoS Biology, 20(1), p.e3001476.
Gilbert, D., 2006. Stumbling on Happiness. New York: Knopf.
Glimcher, P.W., 2010. Foundations of Neuroeconomic Analysis. Oxford University Press.
Kahneman, D., Wakker, P.P. and Sarin, R., 1997. Back to Bentham? Explorations of experienced utility. The Quarterly Journal of Economics, 112(2), pp.375-406.
Kant, I., 1785. Groundwork of the Metaphysics of Morals. Translated and edited by M. Gregor, 1998. Cambridge: Cambridge University Press.
Kringelbach, M.L. and Berridge, K.C., 2010. The functional neuroanatomy of pleasure and happiness. Discovery Medicine, 9(49), p.579.
Kundera, M., 1984. The Unbearable Lightness of Being. Translated from Czech by M.H. Heim, 1984. London: Faber & Faber.
Montague, R., 2007. Your brain is (almost) perfect: How we make decisions. Penguin.
Montague, P.R., Dayan, P. and Sejnowski, T.J., 1996. A framework for mesencephalic dopamine systems based on predictive Hebbian learning. Journal of Neuroscience, 16(5), pp.1936-1947.
Schultz, W., 2016. Dopamine reward prediction-error signalling: a two-component response. Nature Reviews Neuroscience, 17(3), pp.183-195.
Schultz, W., Dayan, P. and Montague, P.R., 1997. A neural substrate of prediction and reward. Science, 275(5306), pp.1593-1599.
Sutton, R. and Barto G., 2018, Reinforcement Learning, second edition: An Introduction. MIT Press
The idea that happiness is somewhat mysterious has often been expressed. In his Groundwork of the Metaphysics of Morals, Immanuel Kant wrote:
The concept of happiness is such an indeterminate concept that, although every human being wishes to attain this, he can still never say determinately and consistently with himself what he really wishes and wills. - Kant (1785)
More recently, Psychologist Dan Gilbert stressed in his book Stumbling on Happiness that we often do not know what will make us happy and can find out that some things make us unexpectedly happy. I discussed previously why we are often mistaken about what will make us happy in the future.
I highly recommend the fascinating documentary about AlphaGo and its matches against Lee Sedol.
During training, the program had to try new moves that didn’t initially have the highest expected values in order to explore new possibilities. Sometimes, these moves led to better outcomes, and the program updated its values accordingly.
For mathematically inclined readers: this approach is Temporal Difference Learning. Consider the value of an action A in a state S at period t as Q. The Q learning algorithm, one of the possible TD approaches, revises the estimated value of this action after experiencing a reward R following a choice A in state S in the following way:
After making a decision A and experiencing it, the prediction error is the difference between the sum of this immediate reward and the expectation of future rewards (assuming the program will make the best decisions next). Future rewards are discounted with a parameter gamma<1. See Sutton and Barto (2018, chap. 6).
I should note that, while this is my preferred view based on the evidence I have seen, the role of dopamine neurons in explaining hedonic feelings is still debated. See Berridge and Kringelbach (2015) for a different view, and Schultz (2016) and Dayan (2022) for discussions of the theory in relation to recent empirical evidence. Feelings of “contentment” or general satisfaction may possibly come from different neural systems. I will discuss these in a future post.
Note that, using that perspective, it is not difficult to explain why our tastes are socially influenced. The conditions to get primary rewards differ across societies. Hence, the social activities that people like and enjoy, secondary rewards, may vary across cultures. This does not mean that happiness is arbitrarily socially constructed. Different societies simply have different characteristics and different rules of social games—social norms—to obtain primary rewards.
Here is how Read Montague describes it in his great book Your Brain is (Almost) Perfect:
the most powerful learning algorithm on this planet [is] natural selection. Variation lets biological systems explore alternative solutions, selection provides the feedback, and storage allows the system (the natural world) to retain the solutions that worked. The backdrop for this algorithm is the real world, where the harsh demands of survival set standards for what consitutes successful solutions. - Montague (2007)
This might sound like a far-fetched idea, but it is not. Many machine learning algorithms use an evolutionary process to calibrate their parameters: producing many different versions of the algorithm, selecting only the most performing ones, then generating new variations of these best algorithms, selecting the most performing ones among them, and so on. You can find many examples on YouTube of this principle being applied to train algorithms (here for instance to train cars on a game race track).
Really interesting as usual. But the algorithm can’t be as simple as ‘repeat what gives pleasure’. Wasn’t that the big takeaway from Damasio’s work? That we can lose half our brains & make decisions that are proximately optimal - but hopelessly non strategic for longer term decisions? The ability to defer gratification is an important feature of choosing optimal pathways. That may mean risking getting no primary reward (the marshmallow or whatever) in order to get two later. Or taking a pathway that promises pain for uncertain but tasty rewards. For me, depression is a psychological stranding where no pathways seem to be available. But if I recall, this helplessness is not ‘learned’ - it’s the standard operating system for organisms confronted with aversive situations? And it messes up the reward / learning system by over emphasising errors. I’m not sure how a machine would respond to aversiveness? Would it keep trying new pathways until it ran out of energy or would it also adopt a helplessness & throw in the towel after a sub optimal number of attempts? Also, as I’ve previously commented, I find the really fascinating bit is the poorly calibrated people. People with super optimism bias who perceive far more pathways than are available and keep searching for them. But I think these people are also far more likely to get stranded / depressed, because they’re poorly calibrated. Which might explain higher levels of depression among entrepreneurs, sports stars etc; Musk is the perfect example of the massively non-calibrated individual cycling through mood states as he’s forever exploring adaptive pathways. Anyway, as always, a thought provoking post.
Thanks for the overview. I tend to think that these things are really multilayered in our brains.
For example: depression is on a layer above the dopamine actions on neurons and apply a modulation on the overall happiness processing. That is just my intuition from first-hand experience with depression.