The intended third and final post on my series on how to improve debates in the public sphere became long enough that it warranted two posts. The first one argued that we could in principle improve social media platforms by rewarding content that is more consensual—not in the sense that everybody likes it but in the sense that people across partisan lines find it convincing. This post examines how this could be implemented in practice.
The concerns over social media have generated a lot of discussion about what should be done. Some argue for more content moderation, while others suggest that algorithms should be regulated to prevent leading users into rabbit holes of polarising content. In these discussions, users are typically implicitly considered passive receptacles of social media content.
However, this content does not fall from the sky. If users are exposed to bad content on social media (e.g., conflictual, polarising, bad faith), it’s because it is produced by other users in the first place. An important question for improving social media is how to foster better content creation. Not asking this question assumes that bad content will always exist, focusing only on the transmission of content. But this assumption is incorrect. We know that users produce content as a function of how it is rewarded on social media. From an economic perspective, the question becomes “can we shape users’ incentives on social media platforms, to motivate them to contribute better content?”
Today, for many economists, economics is to a large extent a matter of incentives. - Laffont and Martimort (2009)
The incentives of users are primarily the engagement and social recognition (e.g., likes, reposts) they get from posting. In my previous post, I argued that a practical criterion for identifying good content is its likelihood to appeal broadly across partisan lines. In this post, I discuss whether and how social media rewards can be structured to motivate users to strive to produce such content.
The drawbacks of thumb-ups only feedback
On many social media platforms, like Twitter, Facebook, Bluesky, feedback from other users primarily takes the form “thumb-up” options. Once you post something, others can express their approval by clicking on a “like” button or reposting it to their audience.
From the point of view of the social media platform, choosing this type of possible feedback seems, at first sight, reasonable. It allows only positive feedback which is good for the users’ feelings.1 It can also be seen as a kind of vote of support, akin to how decisions are made in a democracy.
In terms of incentives, does such a “thumb-up” approach foster consensual content? Readers familiar with political science might at this point think of the Hotelling-Downs result: voting to select political content can incentivise those offering this content to aim for moderate views, which are more likely to attract a majority of votes.
This result is often illustrated with the analogy that on a beach, two ice cream sellers would end up both located at the very centre, each receiving 50% of the customers. The length of the beach can be thought of as the left-right political dimension and the ice cream sellers are akin to providers of political options. The only stable situation2 is when both providers are in the middle, with no incentive to move in an attempt to capture some of the other provider’s customers.
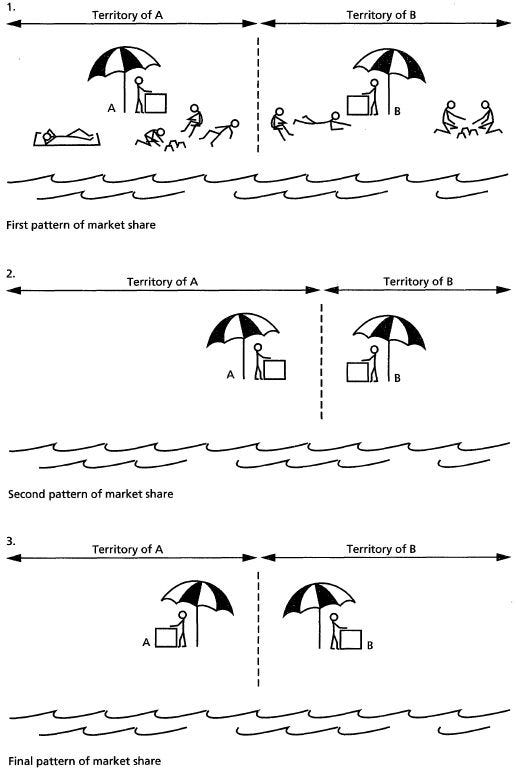
But this result holds only if there are just two providers. With more providers, there may be no stable pattern, or they may choose positions away from the centre. Moreover, it assumes that everyone buys the closest option. In reality, if the ice cream seller is too far from your location on the beach, you might give up on the idea of having ice cream altogether. Similarly, in the political realm, if no politician aligns closely with your positions, you might opt not to vote for anyone (abstention). These deviations from the assumptions underlying the Hotelling-Downs model can lead to polarisation in a political setting (Jones et al., 2022).
If the political space is polarised and divided into strongly opposed subgroups, those supplying political content may receive more attention and more “likes” when addressing specific political subgroups, rather than trying to reach a broad audience. In such scenarios, the thumb-up system of social media can incentivise catering to echo chambers over aiming for broad consensus.
Furthermore, the thumb-up system can create perverse incentives. Users may be incentivised to create engagement even if most reactions are negative. You get more likes if you write a post liked by 200 people and disliked by 1000 people than if you write one liked by 20 and disliked by 10. This fact can motivate some users to express extreme views that provoke and attract the attention of those opposed to them, or they might engage aggressively with users who have a large audience intentionally. This strategy of “clashing”, and “trolling” to create “buzz” aligns with Barnum’s dictum “There’s no such thing as bad publicity”.
Adding a thumb-down feedback: Reddit
An alternative to the thumb-up voting system is to offer users the option to upvote and downvote contributions. This is the case on platforms like Reddit and Hacker News.
In a polarised political space, users who strongly disagree with a post have the option not just to ignore it but also to negatively impact its visibility by downvoting it.3 Downvoted posts are given lower priority in being shown to other users. This mechanism is likely to reduce incentives for posting extremely polarising takes. For instance, while an outlandish post on Twitter may generate outrage and engagement, increasing the visibility of the poster, the same post on Reddit would likely be downvoted to oblivion.
The following figure illustrates the idea that with an upvote/downvote system, posters may maximise their recognition by aligning their content more closely with the average views of the online community.
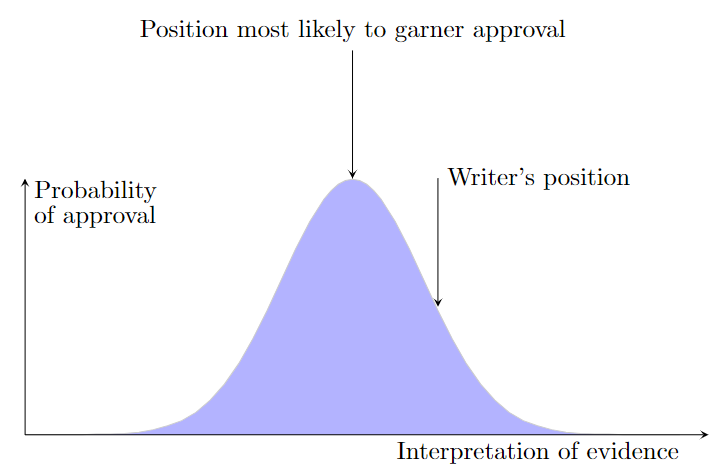
I conjecture that this system may incentivise contributors to submit more consensual and therefore higher-quality content. This is an empirical claim. Whether it holds true in practice would depend on the distribution of political views on social media and the factors leading to upvoting or downvoting. A definitive test of this idea is elusive, but a recent study comparing Twitter and Reddit in their effectiveness for news updates found that information was more rapidly accessible on Reddit and that “Reddit comments would be more suitable to gain additional knowledge on matters related to an event” (Priya et al., 2019).
Another justification for the downvote option is that some users are often drawn to poor-quality content out of curiosity (e.g. the “you won’t believe what happens next” type of posts). For instance, Robertson and colleagues (2024) argue that our curiosity is attracted to potential “threats” like violence, anger, and social outrage. Upon inspection, users may prefer not to see the same content again, but algorithms may simply record that the content succeeded in attracting their attention.
Adding an accessible mechanism that lets users say “I don’t want to see content like this” could substantially reduce people’s exposure to unwanted threatening stimuli that an attentional proxy might promote. - Robertson et al. (2024)
Rewarding reaching consensus via arguments: Wikipedia
One crowdsourced platform generally lauded for the quality of its content is Wikipedia. It would be naive to think that Wikipedian contributors do not have agendas and political preferences (Neff et al., 2013). Wikipedia’s solution to generate quality content from users with potentially slanted motivations involves a tightly guided form of discursive democracy. Contentious content leads to long and protracted discussions with arguments and counterarguments. The outcome tends to incorporate evidence from different perspectives. Decisions emerge through local consensus, voting among users, and occasionally, interventions by contributors with higher moderation rights. These rights are granted based on the voting of a community of contributors, who consider the user’s contribution record.
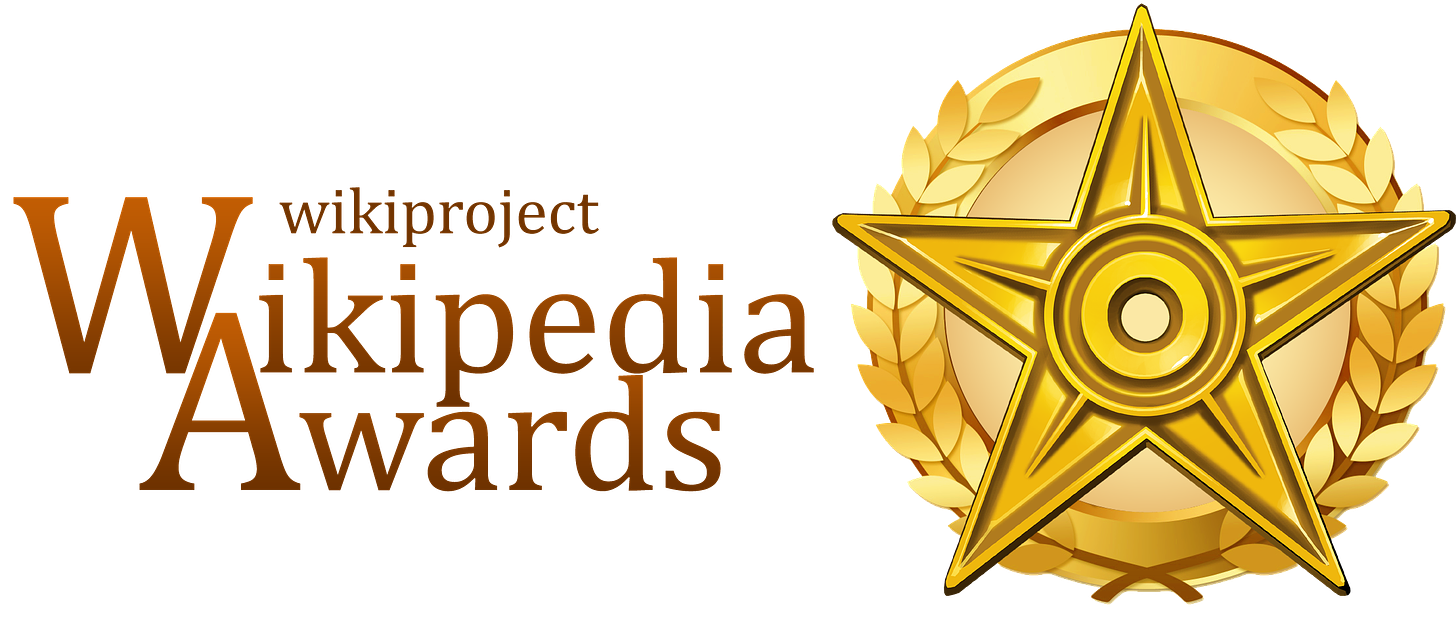
The ability to constructively engage with contributors of varying views while adhering to the platform’s principles for evidence is rewarded with symbolic rewards and higher editorial rights in the community. These rewards create a ladder of contributor status that incentivises Wikipedia contributors. This process to grant these rewards likely encourages the production of more consensual than extreme content.4 The drawback of this system is that it is highly work-intensive, requiring much more time and involvement from contributors than the brief interactions typical on social media. Consequently, it is unlikely to be a model generalisable to other social media platforms where users’ involvement with any given post is short-lived.
Identifying a common denominator algorithmically - Twitter community notes
Social media use algorithms to prioritise or downplay content. Modern data science techniques make it possible for these algorithms to analyse the content of users’ posts.
We can design those algorithms for social good; we can design them to support deliberation and improve the accuracy of people’s beliefs. - Burton (2023)
Ideally, a social platform would want a quick and automated way to identify the consensual nature of contributions to incentivise them. A notable example is the algorithm used by Twitter to determine the quality of its “community notes”. Community notes are annotations added by viewers to a tweet, typically offering corrections, contradictory evidence, or useful context.
In a polarised political environment, the challenge is to ensure that community notes are of high quality and reflect available evidence rather than subjective and partisan views. With the vast number of community notes submitted, this challenge should ideally be tackled using crowdsourcing and an algorithm, rather than relying on Twitter employees’ input.
Twitter’s solution involves allowing interested individuals to sign up to write notes and provide feedback on submitted community notes. Crucially, the algorithm doesn’t just select notes that gather majority support, but those “rated helpful by people from diverse perspectives”. Twitter is transparent about its algorithm, which identifies what is driven by users’ idiosyncratic preferences and what is driven by a common perception of a note’s usefulness. This common perception, shared across users with differing views, is what determines which notes are published.
Twitter uses the same type of data analysis used by Netflix to recommend movies. While Netflix focuses on identifying viewers’ different preferences, Twitter concentrates on commonalities among users.5
Going one step further, Twitter then uses this scoring system to evaluate the users who rated the notes. Users who consistently rate helpful notes as helpful are themselves deemed helpful. The notes’ scores from this subset of “helfpful” users are those used to identify the most beneficial notes. This effectively screens out unhelpful or partisan raters. The outcome of this process has been generally well-received, with community notes being praised as a useful feature on Twitter.6
Measuring a poster’s centrality in the network - Google PageRank
Another digital platform renowned for successfully extracting useful information from a vast array of content with variable quality is Google. The cornerstone of Google’s approach is the PageRank algorithm (Brin and Page, 1998). The underlying concept of this algorithm is straightforward: pages receiving more links are likely to be of higher quality, and consequently, the links they provide are also likely to be more valuable.7
The PageRank algorithm yields results by recursively applying that principle. Firstly, assign scores to pages based on their incoming links: pages that receive more links get a higher score. Secondly, weight each page's outgoing links with the score of that page, making links from popular pages more valuable than those from less popular ones. Then, restart this process: recalculate the scores for all pages using these newly weighted links, and then use these updated scores to reweight their outgoing links. This process is repeated until it converges to stable scores for each page. These scores give more importance to pages that receive more links from pages that receive more links from pages that receive more links…
This solution is a measure of centrality in the network called eigenvector centrality.8 This measure of centrality may be more conducive to identifying quality content because it gives greater weight to people who receive recognition from people also receiving recognition. The effect of using eigenvector centrality in a polarised network is I think a priori unclear. In practice, it may help reduce polarisation by rewarding influencers who are consensual enough to receive approval from influencers of the different clusters in the network. I illustrate this with the figure below which compares the weight of different content providers in a network as a function of the number of links received (left) and as a function of eigenvector centrality (right). The Google-like approach gives relatively more weight to the central provider.
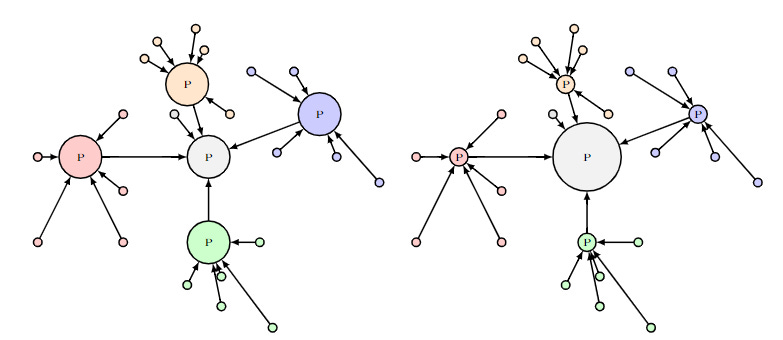
A measure of social recognition on digital platforms using this type of centrality measure may incentivise content providers to be more consensual to get support from large users from across the network.
What’s the practical point of this discussion?
It's one thing to theorise about ways to improve social media, but quite another to get these solutions implemented in practice. Social media platforms are organised by private companies and as pointed out by Cohen and Fung:
Private companies, their rhetoric aside, are often in the business of advertising and attention, not providing reliable, relevant information and enabling public communication. - Cohen and Fung (2021)
Policy making
One possibility is to use these ideas to inform the regulation of social media. Simons and Gosh (2020) have argued for regulating algorithms that shape how people find content.
The design of algorithms in internet platforms has become a kind of public policymaking. The goals and values built into the design of these algorithms, and the interests they favor, affect our society, economy, and democracy. […] Each time Facebook and Google make decisions about these machine learning algorithms, they exercise a kind of private power over public infrastructure. - Simons and Gosh (2020)
This idea of regulation is present in policy-making circles. The European Council (2020) in its “conclusions on safeguarding a free and pluralistic media system” recommended considering the impact of social media algorithms on users’ information when designing media policy.
Informing social media companies about best practice
While private companies are driven by profit, in some cases this concern can lead to socially positive practices.
Business actors [may be] willing to steer their activities toward responsible practices, aligning on international sustainability indicators and shifting the media ecosystem in a healthier direction, on the basis of self-regulation. - Giannelos (2023)
The New York Times reported in 2021 on the internal tensions within Meta regarding engagement maximisation versus reducing harmful content. Meta surveyed users about posts they found “good for the world” or “bad for the world.” They found that “high-reach posts — posts seen by many users — were more likely to be considered ‘bad for the world’”. Consequently, they implemented an algorithm that deprioritised such content. However, observing a decrease in Facebook usage, the company revised the algorithm to not demote “bad” content as strongly, balancing the reduction of harmful content with user engagement. A similar tension between promoting “responsible” content and fostering engagement has been reported at Youtube.
There are potential benefits for companies in promoting quality content. While low-quality/high-engagement content may boost short-term engagement, users may eventually become disillusioned and reduce their interaction. High-quality content can build a platform's reputation, offering long-term benefits (Gentzkow and Shapiro, 2008). Focusing on short-term engagement with low-quality content might damage a platform's reputation and long-term user engagement. For that reason, it may be in the interest of social media platforms to enhance the quality of the content they present.
Conclusion to this series: Science as a model
In this series of three posts, I have explored ways to enhance public debate, acknowledging that most participants are more focused on finding the best arguments than seeking the truth. To conclude, let’s compare these suggestions with the features of the most successful social setting for advancing human knowledge: science.
Popper described science as an ideal marketplace of ideas:
We choose the theory which best holds its own in competition with other theories; the one which, by natural selection, proves itself the fittest to survive. - Popper (1934)
However, this view is often criticised for being overly idealistic. The physicist Max Planck famously stated:
A new scientific truth does not triumph by convincing its opponents and making them see the light, but rather because its opponents eventually die, and a new generation grows up that is familiar with it. - Planck (1950)
While Planck's view may be too cynical, and scientists do change their minds, they also have incentives, agendas, preferences, and biases. The recent replication crisis in behavioural sciences has exposed how incentives to publish large quantities of papers with surprising results led to many dubious publications.
Despite these human flaws, science has succeeded in fostering the acquisition and accumulation of knowledge. The characteristics of science as a social setting offer insights into what’s needed to succeed in the challenge of pulling ourselves by our hair outside of nonsense discussions.
1. Free entry of ideas:
Science thrives on free inquiry, with no question or answer being off-limits, though certain methods of inquiry can be. This is in line with my reasoned case for freedom of speech in the public sphere.
2. Controlling conflicts of interests
While all ideas are welcome, scientific institutions are wary of the conflicts of interest which may mislead the processes of intellectual inquiry. Journals, typically independent from governments and private firms, require scientists to declare any third-party benefits. This is in line with my discussion of the regulation of the marketplace of ideas to limit the overdue influence of external actors with motives other than promoting the best ideas.
3. Rewards favouring consensual views
The social rewards in science encourage scientists to aim for more consensual takes. The peer review system, acting as gatekeeping for scientific publications, subjects a paper to the scrutiny of unrelated peers with expertise in the field. Peer review is often criticised as being counterproductive. For instance, it is commonly said that scientific papers do not improve much through the peer review process. But this take misses a critical aspect of peer review: its main impact is likely not to improve scientific papers after submission, but to force scientists to write their articles before submission in a way that could be consensual9 for most reviewers (sampled from the pool of experts in the field). It is the incentive effect of peer review that is likely the most important in influencing scientific writing.
Finally, there is the social prestige associated with publishing in leading scientific journals. Scientists have a reasonably shared understanding of what are the “best” journals in their field. This measure is often imperfectly related to impact factors (average number of citations received by the journal’s articles). Instead, I think that scientists’ intuitive ordering of journals is more similar to Google PageRank’s algorithm: technical journals with low citations but being cited by other leading journals are more likely to be considered highly. Following this intuition, there is a website that provides a ranking of scientific journals using this method.
Building a public sphere where the best ideas prevail is challenging because it is a socially desirable outcome that does not align with individuals’ motivations for participating in public discussions. The specific solutions crafted and implemented in science offer us a model to consider how to improve public discussions. Discussions on social media cannot replicate the highly work-intensive aspect of scientific discourse, but we can extract some key principles to guide us in shaping digital platforms.
This is the last post in the series on how to improve the quality of public debates. In the next posts, I’ll explore new topics in psychology and game theory.
References
Brin, S. and Page, L., 1998. The anatomy of a large-scale hypertextual web search engine. Computer networks and ISDN systems, 30(1-7), pp.107-117.
Burton, J.W., 2023. Algorithmic Amplification for Collective Intelligence. https://knightcolumbia.org/content/algorithmic-amplification-for-collective-intelligence
Cohen, J., Fung, A., Bernholz, L., Landemore, H. and Reich, R., 2021. Democracy and the digital public sphere. Digital technology and democratic theory, pp.23-61.
European Council, 2020. Council conclusions on safeguarding a free and pluralistic media system. https://eur-lex.europa.eu/legal-content/EN/TXT/?uri=CELEX%3A52020XG1207%2801%29
Gentzkow, M. and Shapiro, J.M., 2008. Competition and Truth in the Market for News. Journal of Economic Perspectives, 22(2), pp.133-154.
Giannelos, K., 2023. Recommendations for a Healthy Digital Public Sphere. Journal of Media Ethics, 38(2), pp.80-92.
Hsiao, Y.T., Lin, S.Y., Tang, A., Narayanan, D. and Sarahe, C., 2018. vTaiwan: An empirical study of open consultation process in Taiwan.
Jones, M.I., Sirianni, A.D. and Fu, F., 2022. Polarization, abstention, and the median voter theorem. Humanities and Social Sciences Communications, 9(1), pp.1-12.
Laffont, J.J. and Martimort, D., 2009. The theory of incentives: the principal-agent model. In The theory of incentives. Princeton University Press.
Mehlhaff, I.D., 2023. Political Argumentation and Attitude Change in Online Interactions. Working paper.
Neff, J.J., Laniado, D., Kappler, K.E., Volkovich, Y., Aragón, P. and Kaltenbrunner, A., 2013. Jointly they edit: Examining the impact of community identification on political interaction in Wikipedia. PloS one, 8(4), p.e60584.
Planck, M., 1950. Scientific autobiography: And other papers. William and Northgate.
Popper, K., 1934. The logic of scientific discovery.
Priya, S., Sequeira, R., Chandra, J. and Dandapat, S.K., 2019. Where should one get news updates: Twitter or Reddit. Online Social Networks and Media, 9, pp.17-29.’Raspe, R. E., 1785. Baron Munchausen's Narrative of his Marvellous Travels and Campaigns in Russia.
Robertson, C., del Rosario, K., Rathje, S. and Van Bavel, J.J., 2023. Changing the incentive structure of social media may reduce online proxy failure and proliferation of negativity. Preprint (forthcoming in Brain and Behavioral Sciences).
Simons, J. and Ghosh, D., 2020. Utilities for democracy: Why and how the algorithmic infrastructure of Facebook and Google must be regulated. Brookings website.
Small, C., Bjorkegren, M., Erkkilä, T., Shaw, L. and Megill, C., 2021. Polis: Scaling deliberation by mapping high dimensional opinion spaces. Recerca: revista de pensament i anàlisi, 26(2).
Smith, M.A., Himelboim, I., Rainie, L. and Shneiderman, B., 2015. The structures of Twitter crowds and conversations. Transparency in Social Media: Tools, Methods and Algorithms for Mediating Online Interactions, pp.67-108.
Wojcik, S., Hilgard, S., Judd, N., Mocanu, D., Ragain, S., Hunzaker, M.B., Coleman, K. and Baxter, J., 2022. Birdwatch: Crowd wisdom and bridging algorithms can inform understanding and reduce the spread of misinformation. arXiv preprint arXiv:2210.15723.
Yang, H.L. and Lai, C.Y., 2010. Motivations of Wikipedia content contributors. Computers in human behavior, 26(6), pp.1377-1383.
YouTube decided to make the count of its dislike button invisible to protect the well-being of its creators. In a statement about the decision, the company said it was meant to “help better protect our creators from harassment, and reduce dislike attacks”.
This stable situation is the Nash equilibrium of this game.
To limit the downvote pile-ons, both Reddit and Hacker News display only the difference between upvotes and downvotes, and only if it is positive. A heavily downvoted post will therefore show a score of 0, similar to a post without any engagement. Hacker News also limits the downvoting option to users who have already contributed substantially upvoted content in the past.
The subreddit r/ChangeMyView also rewards posters for convincing others, who then grant a 'delta' to the poster who changed their mind. Mehlhaff (2023) found that while most contributors do not change their mind, argumentative exchanges can lead to a change of mind in some users.
For the mathematically minded reader:
Netflix’ approach, described here and here consists in considering that a movie has a number of characteristics (e.g. action, drama, long, European…). Each movie can therefore be represented by a vector of characteristics f_m. Users have preferences for each of these characteristics represented by a vector of parameters f_u, indicating the sign and magnitude of preference the user’s preference for each characteristic (e.g. dislikes a bit action movies, likes thrillers, loves British movies...). A user’s idiosyncratic preference for a given movie with a vector fm is therefore the cross-product f_m . f_u.
A working paper from the Twitter team suggests that Twitter estimates the following model (Wojcik et al., 2022):
With a user rating of a note being the reflection of a general tendency to give good or bad ratings (mu) the propensity of the user to give good or bad ranging (i_u), the propensity of this specific note to get good or bad ratings (i_n) and the user idiosyncratic preference for this note (f_n . f_u). Twitter only retains the parameter i_n, used as a score of general usefulness measured across users with different viewpoints.
They illustrate this approach with this figure, using only one dimension (factor) to represent the characteristics of the notes. The note intercept is the coefficient i_n.
Another example of prioritisation of consensual content is the approach followed by Polis, an online platform designed for argumentative deliberations. People are invited to provide arguments for or against a position and to upvote/downvote existing arguments. These answers are used to identify political groups and their leading arguments. Arguments that are consensual across groups are prioritised to be shown to users. This platform was used by the Taiwanese government for consultations about the regulation of Uber and Airbnb (Small et al., 2021; Hsiao et al., 2018).
You may recognise the same logic in Twitter notes’ algorithm.
For the mathematically minded reader:
Consider a set of pages with links between them. Each page i has n_i outgoing links. Let A be the matrix of links from each page to each page, with each link having a weight 1/n_i in that matrix. The PageRank scores are given by the unique eigenvector of the eigenvalue 1 of the matrix A. By the Perron-Frobenius theorem, the recursive process—starting with a vector v giving each page an equal weight—Av, A2v, A3v… converges to this eigenvector. For a detailed explanation: link.
I stress again that I use the word “consensual” not to refer to ideas that should be liked by everybody, but to ideas that should be convincing to most people, based on the evidence presented and their logical coherence.
Only having 'liking' or 'liking and disliking' is inherently polarising. We need more options.
*funny*, *great parody*, *made me think*, *I didn't know that*, *well-argued*, *well-argued but I still think you are wrong*, *your bias is showing*, *preaching only to the converted*, *rude*, *complete nonsense*, *pure emotional manipulation* etc.